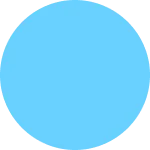

AI Threat Modeling: Steps to Implement in Your Organisation
In the digital landscape of 2024, protecting data is more critical than ever. Cyber threats continue to evolve, becoming more sophisticated and harder to detect. This is where AI threat modelling comes into play. By leveraging artificial intelligence, we can predict and counteract potential threats before they cause harm.
Implementing AI threat modelling in an organisation isn't just about adopting a new technology; it's about changing how we approach cybersecurity. This approach allows us to identify weaknesses and anticipate attacks with a precision that traditional methods cannot achieve. Our goal should be to integrate AI into every layer of our security strategy, providing a robust defence against the constantly changing threat landscape.
Embarking on the journey to implement AI threat modelling can seem daunting, but understanding the basic concepts and steps involved can simplify the process.
Understand the Basics of AI Threat Modeling
AI threat modelling is a process that helps us identify, evaluate, and mitigate potential security threats using artificial intelligence. This method is pivotal as it allows us to predict and act on possible security issues before they can cause harm. AI threat modelling involves the use of algorithms and machine learning to analyse large amounts of data, detecting patterns that may indicate a threat.
In 2024, the importance of AI threat modelling has grown significantly due to the evolving nature of cyber threats. Traditional methods, which often rely on reactive measures, are no longer sufficient. AI threat modelling, on the other hand, provides a proactive approach, continuously learning from new data to improve its threat detection capabilities. By understanding how AI threat modelling works, we can better prepare and protect our digital environments.
Key Steps to Implement AI Threat Modeling
Implementing AI threat modelling involves several key steps to ensure its effectiveness. First, we need to define the scope of our threat modelling. This includes identifying the assets we want to protect and understanding the potential threats they may face. Clearly defining the scope helps focus AI efforts on the most critical areas.
Next, gathering relevant data is essential. The AI systems need high-quality, comprehensive data to learn and make accurate predictions. This data could include past security incidents, network logs, and any other information that reflects the state of our digital environment.
Once we have the data, the next step is to train the AI models. This involves feeding the data into the AI system and allowing it to learn from patterns and anomalies. Continuous training and updating ensure that the AI stays up-to-date with the latest threat landscape and can adapt to new threats as they emerge.
Finally, integrating the AI threat modelling into our existing security framework is crucial. This ensures that the insights and alerts generated by the AI are utilised effectively to enhance our overall security posture. Regular monitoring and reviewing the performance of the AI models also help in refining the threat modelling process.
Common Challenges and How to Overcome Them
Implementing AI threat modelling comes with several challenges. One significant challenge is the quality of data. Poor or biased data can lead to incorrect threat predictions and ineffective defences. To overcome this, we must ensure that the data used for AI training is diverse, accurate, and up-to-date. Regular data audits and cleaning processes can help maintain data integrity.
Another challenge is the integration of AI systems with existing security infrastructure. Combining new AI technologies with legacy systems can be complex and may require significant adjustments. We can tackle this by planning phased integration and conducting thorough compatibility tests. Ensuring that our teams are well-trained and supported during this transition is also crucial to smooth implementation.
Best Practices for Sustaining AI Threat Modelling Efforts
To maintain effective AI threat modelling, adhering to best practices is essential. Firstly, ongoing training and education for security teams are vital. As AI technologies evolve, continuous learning ensures that our teams are equipped with the latest knowledge and skills to manage these systems.
Regularly updating AI models and algorithms is another best practice. Cyber threats are constantly evolving, and so should our defences. By keeping AI models current with the latest threat data, we ensure they remain effective against new types of attacks. Scheduled reviews and updates can be part of our regular maintenance routines.
Additionally, collaboration across different departments can enhance the effectiveness of AI threat modelling. Security is a shared responsibility, and insights from various areas of the organisation can help identify and mitigate risks more comprehensively. Regular cross-team meetings and information sharing can foster a collaborative security culture.
Conclusion
AI threat modelling is a powerful tool in our cybersecurity arsenal. By understanding its basics and implementing it effectively, we can protect our data more robustly. Despite the challenges, with the right strategies and best practices, we can sustain our AI threat modelling efforts and stay ahead of emerging threats.
As we navigate the complexities of modern cybersecurity, embracing these advanced technologies is crucial for our defence mechanisms. AI threat modelling not only helps identify potential risks but also provides proactive solutions to mitigate them. By staying informed and adaptable, we can ensure our security measures remain resilient and effective.
Partner with Aristiun to enhance your cybersecurity framework with cutting-edge AI threat modelling solutions tailored to your organisation's needs. Let us help you safeguard your data against the ever-evolving threat landscape of 2024 and beyond.