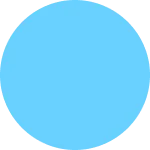

How AI Threat Modeling Protects Data in 2025
As we go into 2025, cyber threats have become more advanced than ever before, making the safety of our data a top priority. One of the most effective ways to guard against these evolving dangers is through AI threat modeling. This powerful tool helps us identify potential security risks before they can cause damage. By predicting where and how attacks might occur, we can prepare better defences and keep sensitive information safe.
AI threat modeling is not just a futuristic concept; it is a practical approach that we can use right now. It allows us to stay one step ahead of cybercriminals by continuously analysing patterns and behaviours within our systems. This means that our data is always under vigilant protection, adapting to new threats as they arise. Whether you're a small business or a large corporation, understanding and implementing AI threat modeling is crucial to safeguarding valuable data in today’s digital landscape.
This article will explore what AI threat modeling is, the necessary steps to implement it, the key benefits it offers, and the best practices for making the most of this technology in 2025. By understanding these elements, we can create a more secure environment for our data and reduce the risk of cyber-attacks.
What is AI Threat Modeling?
AI Threat Modelling is a process that helps us understand and manage potential security risks before they can cause harm. It involves identifying possible threats to our systems and data, evaluating how serious these threats are, and finding ways to protect against them. This approach uses artificial intelligence to analyse data, learn from past incidents, and predict future risks.
Using AI, we can create detailed models of potential threats. These models help us understand how an attacker might try to compromise our systems. By simulating different attack scenarios, AI threat modeling allows us to see where our defences might be weak and how we can strengthen them. This proactive approach helps to keep our data safe and our systems secure.
Steps to Implement AI Threat Modeling
Implementing AI threat modeling involves a series of planned steps that ensure we cover all angles of potential cybersecurity risks. First, we need to identify assets that need protection. These could be databases, network systems, or even specific types of data. Understanding what we need to protect helps us focus our efforts on the most critical areas.
Next, we look at potential threats. This step involves gathering intelligence on possible attack vectors and understanding how these threats could target our assets. Using AI, we can automate this process, allowing for continuous monitoring and analysis. After identifying threats, we analyse vulnerabilities. AI tools help us detect weak points in our cybersecurity defences that attackers might exploit.
Once we’ve identified and analysed threats and vulnerabilities, we need to develop and implement response strategies. These strategies might include updating software, implementing new security protocols, or training staff on cybersecurity practices. Finally, we need to continuously monitor and update our models. As new threats emerge, our AI systems learn and adapt, ensuring our defences remain robust and effective.
Benefits of AI Threat Modeling for Protecting Data
AI threat modeling significantly boosts our data protection efforts in several ways. One of the primary benefits is its ability to provide continuous monitoring. Traditional security measures often involve periodic checks and updates, but AI operates 24/7, constantly scanning for potential vulnerabilities. This round-the-clock vigilance ensures that threats are detected and mitigated in real-time, reducing the risk of data breaches.
Another major benefit is the predictive capability of AI threat modeling. By analysing vast amounts of data, AI can identify patterns that precede security incidents, allowing us to anticipate and address threats before they materialise. This proactive approach is particularly useful in protecting sensitive information, as it helps us stay one step ahead of cybercriminals who are always seeking new ways to exploit weaknesses.
Best Practices for Effective AI Threat Modeling in 2025
To ensure that AI threat modeling is as effective as possible, we should follow some best practices. Firstly, it is crucial to maintain high-quality data. The efficacy of AI is directly tied to the quality of data it processes. Regular audits and updates of data sources help ensure accuracy and relevance, which enhances the AI’s ability to identify genuine threats.
Another best practice is continuous system training. AI systems need regular updates to keep up with the constantly evolving threat landscape. This includes updating algorithms and incorporating the latest threat intelligence. By continuously training our AI systems, we ensure they remain effective against new and emerging threats.
Integrating AI threat modeling into our overall security framework is also essential. AI should complement existing security measures, not replace them. Combining AI with human expertise and traditional security tools creates a layered defence strategy that is robust and adaptable.
Conclusion
AI threat modeling revolutionises how we protect our data by providing continuous monitoring and predictive capabilities. While there are challenges in ensuring data quality and maintaining system updates, following best practices can mitigate these issues. As we embrace the technological advancements of 2025, AI threat modeling will play a crucial role in safeguarding our information.
By implementing these strategies effectively, we're better positioned to tackle the complex challenges of modern cybersecurity. At Aristiun, we specialise in embedding AI-driven security solutions tailored to your unique needs. Let us help you create a secure environment that can withstand the evolving threat landscape. Contact us today to learn how we can fortify your cybersecurity framework through automated threat modeling and other solutions.